Liveness detection technology, also known as facial liveness detection, is a key component of most facial recognition systems today, used to differentiate between a real person and a spoof attack.
Its purpose is to ensure that the biometric data being presented is real (from a live person) and not fake (spoofed images or printed masks). Spoofing attacks are commonly employed by fraudsters to create fake accounts and bypass eKYC processes, among a range of other fraudulent activities.
Highly regulated industries such as BFSI and telco companies face challenges in complying with data protection regulations while actively defending against fraud attempts.
How Does Liveness Detection Work?
Liveness detection is an ID verification technique that detects presentation attacks by identifying the characteristics of a live person. These techniques use algorithms and sensors to identify live biometric data such as faces, fingerprints, and voices.
In facial recognition systems, liveness detection algorithms will analyse subtle facial movements like blinking and head rotation to differentiate live faces from spoofed ones.
To detect facial presentation attacks, liveness detection uses 2D and 3D facial recognition technology, motion tracking, and thermal imaging techniques to scrutinise discrepancies in a person’s face.
There are two types of liveness detection:
- Active liveness detection: This technique requires users to perform specific facial movements. These include actions such as smiling or blinking to prove their liveness.
- Passive Liveness Detection: This technique does not require any input from the user. Instead, it analyses a subject’s face in real-time. It uses artificial intelligence and deep learning algorithms to identify spoofing attempts. Passive liveness checks happen in the background. For instance, a phone recognition system scans the user’s face and detects natural movements like blinking to confirm authenticity.
To learn more about passive and active liveness detection — refer to our dedicated wikipage here
What Techniques Are Involved In The Liveness Detection Process?
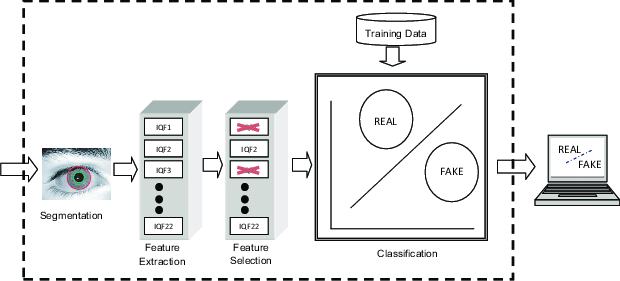
Challenge & Response
Challenge and Response is an example of active liveness detection that requires the user to respond to prompts like blinking, moving the head, smiling, and so on.
The goal of this technique is to detect and block false representations like 2D photographs by having the user prove that they are in fact a live person. The ‘Challenge’ refers to the prompts required to be performed by a user and the ‘Response’ are their movements.
Depth & Motion Perception
In this step, a 3D liveness check is used to map a user’s face to detect presentation attacks. 3D liveness checks tend to be more secure because depth perception is used to great effect in gathering detailed information on a subject’s facial expressions.
The three-dimensional analysis of a subject’s face ensures that 2D spoofing attempts such as a printed photo is detected and countered easily.
Machine Learning/Artificial Intelligence
Algorithmic analysis is used to determine whether a provided sample matches a pre-registered sample. The integration of artificial intelligence and machine learning also automatically recognises variations in an authorised user’s face — such as the growth of facial hair or the presence of glasses.
What Are Presentation Attacks?
Spoofing attacks (also known as presentation attacks) are attempts to bypass biometric authentication systems by presenting manipulated biometric data. This is essentially a form of biometric spoofing that involves exploiting the limitations of a biometric authentication system. This includes using impersonation tools such as a mask or photograph, to trick the system into believing it is a live and authorised user.
There are four types of ways presentation attacks can occur:
- 2D static attacks
- This type of spoofing attack involves presenting a high-quality printed picture of a user’s face to a biometric authentication system.
- 2D dynamic attacks
- Fraudsters can imitate user actions and commit identity theft by displaying a sequence of 2D images or playing them back as a video. This can be carried out on a high-resolution screen, which can fool low-resolution cameras.
- 3D static models, prints, or masks
- In this method, the fraudster employs three-dimensional presentations instead of flat images to commit identity theft. They may use a 3D mask or construct a face model to replicate the likeness of a targeted user.
- 3D dynamic digital doubles and deep fakes
- Similar to 2D Dynamic Attacks, this method uses moving 3D images to spoof user identities. A version of this method involves using a 3D avatar that can convincingly simulate a real face viewed from different angles.
Applications of Liveness Detection In Security
Remote Identity Verification
Liveness detection is used to help digital apps remotely verify their users. Some apps such as banking and carsharing services, require their users to be verified and eligible before they are allowed to use their services.
Liveness detection ensures that a user is physically present at the time of the remote identity verification process to prevent cases of identity fraud.
Remote Customer Onboarding
During the customer onboarding process, liveness detection verifies that the user who is presenting their ID is indeed the same person shown in the ID photo. It also ensures that the user is real and physically present at the time of registration.
Biometric Access Control Systems
Liveness detection allows users to gain instant access to restricted areas or data files without the need to carry access cards or IDs.
To learn more about how facial recognition ties in with access control systems, read our article here
What Are the Challenges Faced During Liveness Detection?
The accuracy of the entire facial recognition process might be affected by low-light conditions. Facial recognition cameras need to sufficiently illuminate a subject’s face to detect and verify their identity properly.
Cameras with low-resolution lenses may also face challenges in detecting the facial profile of a subject. The resulting image capture, with its low quality, might lack the details needed for the liveness detection algorithm to work.
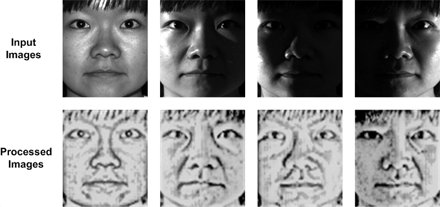
Fortunately, researchers are actively developing built-in image enhancement features to boost the contrast of low-light images artificially. These features also increase the quality (pixel resolution) of an image. The experiment showed impressive results, with an accuracy rate of 76.92% achieved at a brightness level of -80.
Simpler methods involve implementing better-quality cameras and adequate light sources with facial recognition systems.
Bottom Line
Most facial recognition systems rely on liveness detection as a vital component. Liveness detection adds an extra layer of security to the biometric authentication process by ensuring the presence of a real and genuine user during facial recognition.
Many industries are already incorporating liveness detection as a reliable form of biometric authentication measure. The systems used for liveness detection are robust, highly accurate, and capable of effectively screening even the most realistic presentation attacks.